Why Verizon?
The first question that came to mind when the Guardian broke the NSA-Verizon news.
Here’s why I ask:
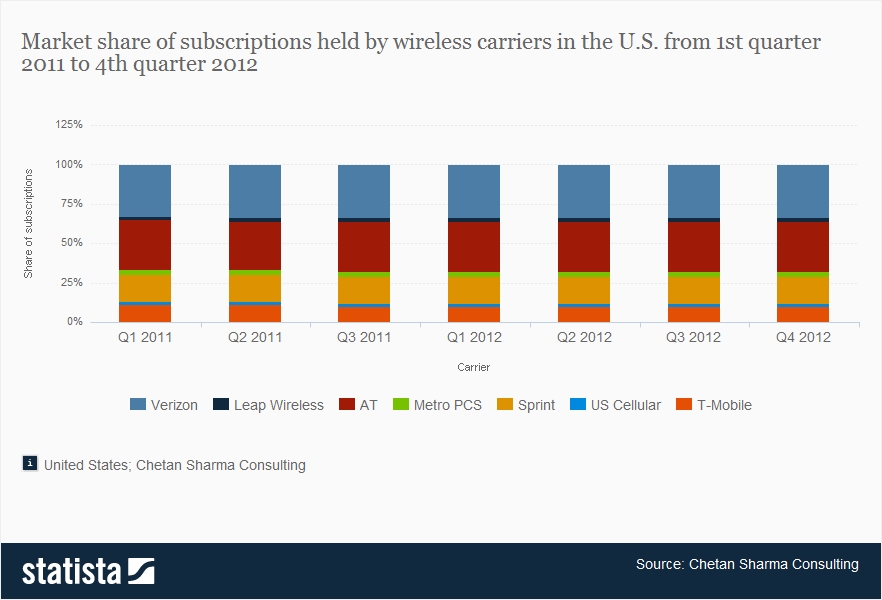
(source: http://www.statista.com/statistics/199359/market-share-of-wireless-carriers-in-the-us-by-subscriptions/)
Verizon over 2011-2012 had only 34% of the cell phone market.
Unless terrorists prefer Verizon for ideological reasons, why Verizon?
Choosing only Verizon means the NSA is missing 66% of potential terrorist cell traffic.
That sounds like a bad plan.
What other reason could there be for picking Verizon?
Consider some other known players:
President Barack Obama, candidate for President of the United States, 2012.
“Bundlers” who gathered donations for Barack Obama:
Min |
Max |
Name |
City |
State |
Employer |
$200,000 |
$500,000 |
Hill, David |
Silver Spring |
MD |
Verizon Communications |
$200,000 |
$500,000 |
Brown, Kathryn |
Oakton |
VA |
Verizon Communications |
$50,000 |
$100,000 |
Milch, Randal |
Bethesda |
MD |
Verizon Communications |
(Source: OpenSecrets.org – 2012 Presidential – Bundlers)
BTW, the Max category means more money may have been given, but that is the top reporting category.
I have informally “identified” the bundlers as follows:
- Kathryn C. Brown
Kathryn C. Brown is senior vice president – Public Policy Development and Corporate Responsibility. She has been with the company since June 2002. She is responsible for policy development and issues management, public policy messaging, strategic alliances and public affairs programs, including Verizon Reads.
Ms. Brown is also responsible for federal, state and international public policy development and international government relations for Verizon. In that role she develops public policy positions and is responsible for project management on emerging domestic and international issues. She also manages relations with think tanks as well as consumer, industry and trade groups important to the public policy process.
- David A. Hill, Bloomberg Business Week reports: David A. Hill serves as Director of Verizon Maryland Inc.
LinkedIn profile reports David A. Hill worked for Verizon, VP & General Counsel (2000 – 2006), Associate General Counsel (March 2006 – 2009), Vice President & Associate General Counsel (March 2009 – September 2011) “Served as a liaison between Verizon and the Obama Administration”
- Randal S. Milch Executive Vice President – Public Policy and General Counsel
What is Verizon making for each data delivery? Is this cash for cash given?
If someone gave your more than $1 million (how much more is unknown), would you talk to them about such a court order?
If you read the “secret” court order, you will notice it was signed on April 23, 2013.
There isn’t a Kathryn C. Brown in Oakton in the White House visitor’s log, but I did find this record, where a “Kathryn C. Brown” made an appointment at the Whitehouse and was seen two (2) days later on the 17th of January 2013.
BROWN,KATHRYN,C,U69535,,VA,,,,,1/15/13 0:00,1/17/13 9:30,1/17/13 23:59,,176,CM,WIN,1/15/13 11:27,CM,,POTUS/FLOTUS,WH,State Floo,MCNAMARALAWDER,CLAUDIA,,,04/26/2013
I don’t have all the dots connected because I am lacking some unknown # of the players, internal Verizon communications, Verizon accounting records showing government payments, but it is enough to make you wonder about the purpose of the “secret” court order.
Was it a serious attempt at gathering data for national security reasons?
Or was it gathering data as a pretext for payments to Verizon or other contractors?
My vote goes for “pretext for payments.”
I say that because using data from different sources has always been hard.
In fact, about 60 to 80% of the time of a data analyst is spent “cleaning up data” for further processing.
The phrase “cleaning up data” is the colloquial form of “semantic impedance.”
Semantic impedance happens when the same people are known by different names in different data sets or different people are known by the same names in the same or different data sets.
Remember Kathryn Brown, of Oakton, VA? One of the Obama bundlers. Let’s use her as an example of “semantic impedance.”
The FEC has a record for Kathryn Brown of Oakton, VA.
But a search engine found:
Kathryn C. Brown
Same person? Or different?
I found another Kathryn Brown at Facebook:
And an image of Facebook Kathryn Brown:
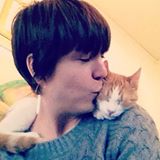
And a photo from a vacation she took:
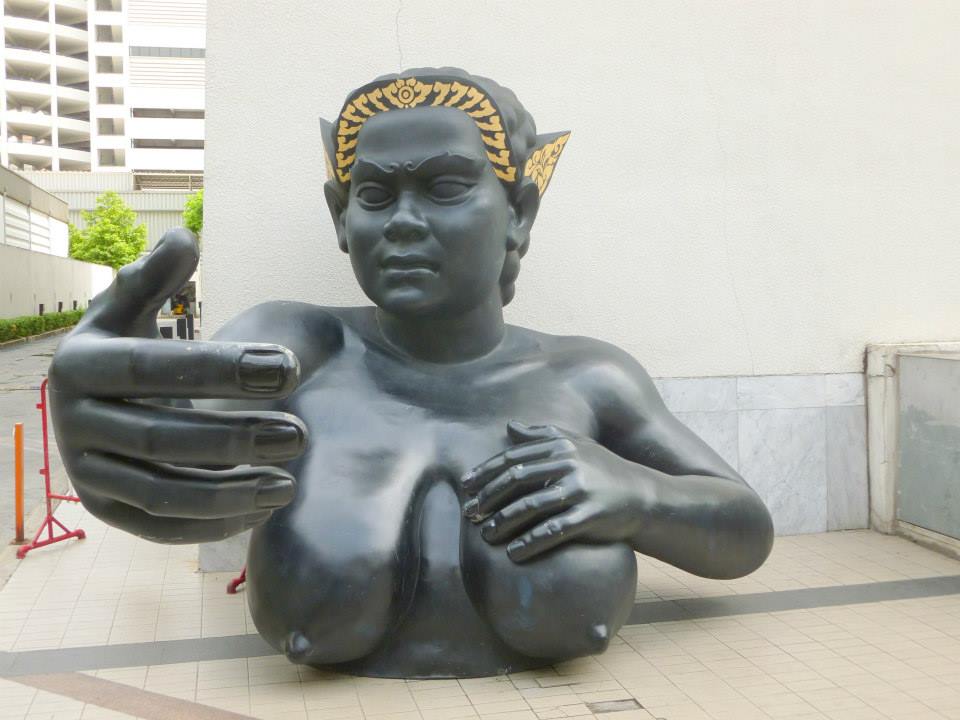
Not to mention the Kathryn Brown that I found at Twitter.

That’s only four (4) data sources and I have at least four (4) different Kathryn Browns.
Across the United States, a quick search shows 227,000 Kathryn Browns.
Remember that is just a personal name. What about different forms of addresses? Or names of employers? Or job descriptions? Or simple errors, like the 20% error rate in credit report records.
Take all the phones, plus names, addresses, employers, job descriptions, errors + other data and multiply that times 311.6 million Americans.
Can that problem be solved with petabytes of data and teraflops of processing?
Not a chance.
Remember that my identification of Kathryn “bundler” Brown with the Kathryn C. Brown of Verison was a human judgement, not an automatic rule. Nor would a computer think to check the White House visitor logs to see if another, possibly the same Kathryn C. Brown visited the White House before the secret order was signed.
Human judgement is required because all the data that the NSA has been collecting is “dirty” data, from one perspective or other. Either is is truly “dirty” in the sense of having errors or it is “dirty” in the sense it doesn’t play well with other data.
The Orwellian fearists can stop huffing and puffing about the coming eclipse of civil liberties. Those passed from view a short time after 9/11 with the passage of the Patriot Act.
That wasn’t the fault of ineffectual NSA data collection. American voters bear responsibility for the loss of civil liberties not voting leadership into office that would repeal the Patriot Act.
Ineffectual NSA data collection impedes the development of techniques that for a sanely scoped data collection effort could make a difference.
A sane scope for preventing terrorist attacks could be starting with a set of known or suspected terrorist phone numbers. Using all phone data (not just from Obama contributors), only numbers contacting or being contacted by those numbers would be subject to further analysis.
Using that much smaller set of phone numbers as identifiers, we could then collect other data, such as names and addresses associated with that smaller set of phone numbers. That doesn’t make the data any cleaner but it does give us a starting point for mapping “dirty” data sets into our starter set.
The next step would be create mappings from other data sets. If we say why we have created a mapping, others can evaluate the accuracy of our mappings.
Those tasks would require computer assistance, but they ultimately would be matters of human judgement.
Examples of such judgements exist, say for example in Palantir product line. If you watch Palantir Gotham being used to model biological relationships, take note of the results that were tagged by another analyst. And how the presenter tags additional material that becomes available to other researchers.
Computer assisted? Yes. Computer driven? No.
To be fair, human judgement is also involved in ineffectual NSA data collection efforts.
But it is human judgement that rewards sycophants and supporters, not serving the public interest.