The Pentagon’s New Artificial Intelligence Is Already Hunting Terrorists by Marcus Weisgerber.
From the post:
Earlier this month at an undisclosed location in the Middle East, computers using special algorithms helped intelligence analysts identify objects in a video feed from a small ScanEagle drone over the battlefield.
A few days into the trials, the computer identified objects – people, cars, types of building – correctly about 60 percent of the time. Just over a week on the job – and a handful of on-the-fly software updates later – the machine’s accuracy improved to around 80 percent. Next month, when its creators send the technology back to war with more software and hardware updates, they believe it will become even more accurate.
It’s an early win for a small team of just 12 people who started working on the project in April. Over the next year, they plan to expand the project to help automate the analysis of video feeds coming from large drones – and that’s just the beginning.
“What we’re setting the stage for is a future of human-machine teaming,” said Air Force Lt. Gen. John N.T. “Jack” Shanahan, director for defense intelligence for warfighter support, the Pentagon general who is overseeing the effort. Shanahan believes the concept will revolutionize the way the military fights.
So you will recognize Air Force Lt. Gen. John N.T. “Jack” Shanahan (Nvidia conference):
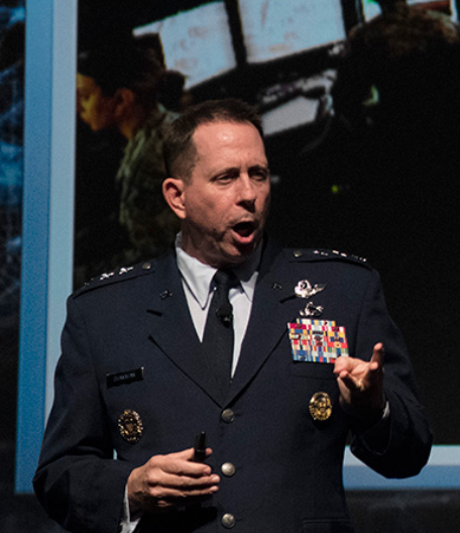
From the Nvidia conference:
Don’t change the culture. Unleash the culture.
That was the message one young officer gave Lt. General John “Jack” Shanahan — the Pentagon’s director for defense for warfighter support — who is hustling to put artificial intelligence and machine learning to work for the U.S. Defense Department.
Highlighting the growing role AI is playing in security, intelligence and defense, Shanahan spoke Wednesday during a keynote address about his team’s use of GPU-driven deep learning at our GPU Technology Conference in Washington.
Shanahan leads Project Maven, an effort launched in April to put machine learning and AI to work, starting with efforts to turn the countless hours of aerial video surveillance collected by the U.S. military into actionable intelligence.
There are at least two market opportunity for adversarial learning. The most obvious one is testing a competitor’s algorithm so it performs less well than yours on “… people, cars, types of building….”
The less obvious market requires US sales of AI-enabled weapon systems to its client states. Client states have an interest in verifying the quality of AI-enabled weapon systems, not to mention non-client states who will be interested in defeating such systems.
For any of those markets, weaponizing adversarial learning and developing a reputation for the same can’t start too soon. Is your anti-AI research department hiring?