Linguistic Mapping Reveals How Word Meanings Sometimes Change Overnight Data mining the way we use words is revealing the linguistic earthquakes that constantly change our language.
From the post:
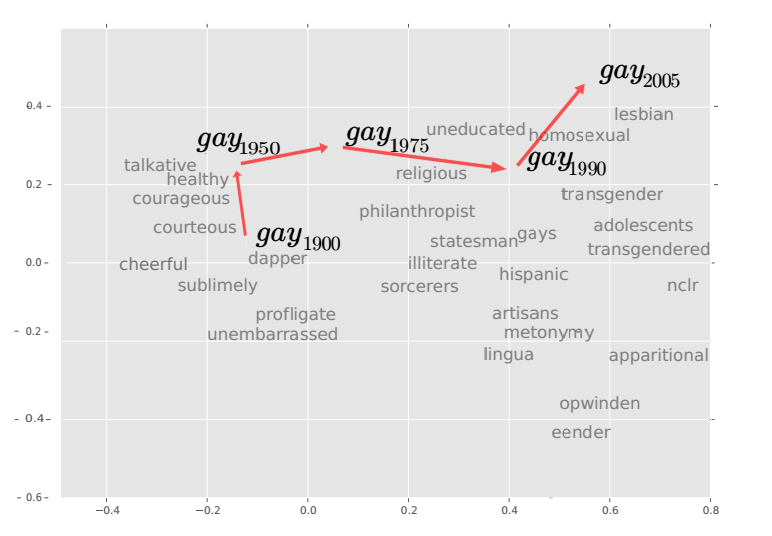
In October 2012, Hurricane Sandy approached the eastern coast of the United States. At the same time, the English language was undergoing a small earthquake of its own. Just months before, the word âsandyâ was an adjective meaning âcovered in or consisting mostly of sandâ or âhaving light yellowish brown colourâ. Almost overnight, this word gained an additional meaning as a proper noun for one of the costliest storms in US history.
A similar change occurred to the word âmouseâ in the early 1970s when it gained the new meaning of âcomputer input deviceâ. In the 1980s, the word âappleâ became a proper noun synonymous with the computer company. And later, the word âwindowsâ followed a similar course after the release of the Microsoft operating system.
All this serves to show how language constantly evolves, often slowly but at other times almost overnight. Keeping track of these new senses and meanings has always been hard. But not anymore.
Today, Vivek Kulkarni at Stony Brook University in New York and a few pals show how they have tracked these linguistic changes by mining the corpus of words stored in databases such as Google Books, movie reviews from Amazon and of course the microblogging site Twitter.
These guys have developed three ways to spot changes in the language. The first is a simple count of how often words are used, using tools such as Google Trends. For example, in October 2012, the frequency of the words âSandyâ and âhurricaneâ both spiked in the run-up to the storm. However, only one of these words changed its meaning, something that a frequency count cannot spot.
…
A very good overview of:
Statistically Significant Detection of Linguistic Change by Vivek Kulkarni, Rami Al-Rfou, Bryan Perozzi, and Steven Skiena.
Abstract:
We propose a new computational approach for tracking and detecting statistically significant linguistic shifts in the meaning and usage of words. Such linguistic shifts are especially prevalent on the Internet, where the rapid exchange of ideas can quickly change a word’s meaning. Our meta-analysis approach constructs property time series of word usage, and then uses statistically sound change point detection algorithms to identify significant linguistic shifts.
We consider and analyze three approaches of increasing complexity to generate such linguistic property time series, the culmination of which uses distributional characteristics inferred from word co-occurrences. Using recently proposed deep neural language models, we first train vector representations of words for each time period. Second, we warp the vector spaces into one unified coordinate system. Finally, we construct a distance-based distributional time series for each word to track it’s linguistic displacement over time.
We demonstrate that our approach is scalable by tracking linguistic change across years of micro-blogging using Twitter, a decade of product reviews using a corpus of movie reviews from Amazon, and a century of written books using the Google Book-ngrams. Our analysis reveals interesting patterns of language usage change commensurate with each medium.
While the authors are concerned with scaling, I would think detecting cracks, crevasses, and minor tremors in the meaning and usage of words, say between a bank and its regulators, or stock traders and the SEC, would be equally important.
Even if auto-detection of the “new” or “changed” meaning is too much to expect, simply detecting dissonance in the usage of terms would be a step in the right direction.
Detecting earthquakes in meaning is a worthy endeavor but there is more tripping on cracks than falling from earthquakes, linguistically speaking.