In Suffix Trees and their Applications in String Algorithms, I pointed out that a subset of the terms for “suffix tree” resulted in About 1,830,000 results (0.22 seconds).
Not a very useful result, even for the most dedicated of graduate students. 😉
A better result would be an indexing entry for “suffix tree,” included results using its alternative names and enabled the user to quickly navigate to sub-entries under “suffix tree.”
To illustrate the benefit from actual indexing, consider that “Suffix Trees and their Applications in String Algorithms” lists only three keywords: “Pattern matching, String algorithms, Suffix tree.” Would you look at this paper for techniques on software maintenance?
Probably not, which would be a mistake. The section 4 covers the use of “parameterized pattern matching” for software maintenance of large programs in a fair amount of depth. Certainly more so than it covers “multidimensional pattern matching,” which is mentioned in the abstract and in the conclusion but not elsewhere in the paper. (“Higher dimensions” is mentioned on page 3 but only in two sentences with references.) Despite being mentioned in the abstract and conclusion as major theme of the paper.
A properly constructed index would break out both “parameterized pattern matching” and “software maintenance” as key subjects that occur in this paper. A bit easier to find than wading through 1,830,000 “results.”
Before anyone comments that such granular indexing would be too time consuming or expensive, recall the citation rates for computer science, 2000 – 2010:
Field |
2000 |
2001 |
2002 |
2003 |
2004 |
2005 |
2006 |
2007 |
2008 |
2009 |
2010 |
All years |
Computer science |
7.17 |
7.66 |
7.93 |
5.35 |
3.99 |
3.51 |
2.51 |
3.26 |
2.13 |
0.98 |
0.15 |
3.75 |
From: Citation averages, 2000-2010, by fields and years
The reason for the declining numbers is that citations to papers from the year 2000 decline over time.
But the highest percentage rate, 7.93 in 2002, is far less than the total number of papers published in 2000.
At one point in journal publication history, manual indexing was universal. But that was before full text searching became a reality and the scientific publication rate exploded.
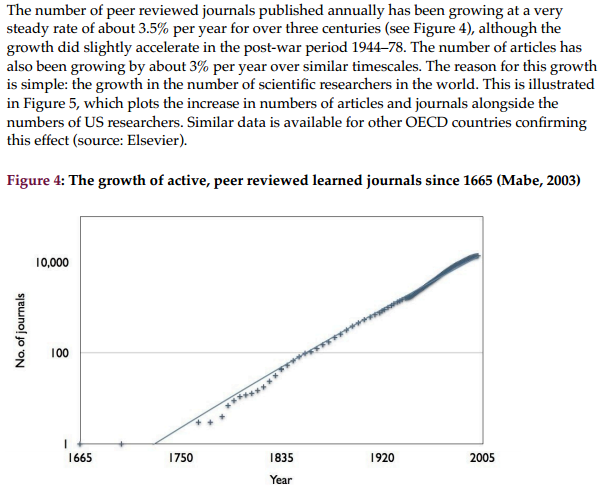
The STM Report by Mark Ware and Michael Mabe.
Rather than an all human indexing model (not possible due to the rate of publication, costs) or an all computer-based searching model (leads to poor results as described above), why not consider a bifurcated indexing/search model?
The well over 90% of CS publications that aren’t cited should be subject to computer-based indexing and search models. On the other hand, the meager 8% that are cited, perhaps subject to some scale of citation, could be curated by human/machine assisted indexing.
Human/machine assisted indexing would increase access to material already selected by other readers. Perhaps even as a value-add product as opposed to take your chances with search access.